1. Настроить репозиторий для docker
```
sudo apt-get update
$ sudo apt-get install \
ca-certificates \
curl \
gnupg \
lsb-release
sudo mkdir -p /etc/apt/keyrings
curl -fsSL https://download.docker.com/linux/ubuntu/gpg | sudo gpg --dearmor -o /etc/apt/keyrings/docker.gpg
echo \
"deb [arch=$(dpkg --print-architecture) signed-by=/etc/apt/keyrings/docker.gpg] https://download.docker.com/linux/ubuntu \
$(lsb_release -cs) stable" | sudo tee /etc/apt/sources.list.d/docker.list > /dev/null
```
2. Установить docker-engine
```
sudo apt-get update
sudo apt-get install docker-ce docker-ce-cli containerd.io docker-compose-plugin
```
3. Загрузить образ с timescaledb
```
docker pull timescale/timescaledb-ha:pg14-latest
```
4.
```
docker run -d --name timescaledb -p 5432:5432 -e POSTGRES_PASSWORD=password timescale/timescaledb:latest-pg14
```
5.
```
docker exec -it timescaledb psql -U postgres
```
6.
```
docker run -d --name timescaledb -p 5432:5432 \
-v /your/data/dir:/var/lib/postgresql/data \
-e POSTGRES_PASSWORD=password timescale/timescaledb:latest-pg14
```
Если self-hosted вариант, тогда надо включить расширение
```
root@db:/etc/apt/sources.list.d# grep timescale /etc/postgresql/14/main/postgresql.conf
shared_preload_libraries = 'timescaledb' # (change requires restart)
```
### Setting up the TimescaleDB extension
1. Создание базы
```
CREATE database example;
```
2. Connect to the database you created:
3. Add the TimescaleDB extension:
```
CREATE EXTENSION IF NOT EXISTS timescaledb;
```
You can check that the TimescaleDB extension is installed by using the \dx command at the psql prompt. It looks like this:
```
example-# \dx
List of installed extensions
Name | Version | Schema | Description
-------------+---------+------------+-------------------------------------------------------------------
plpgsql | 1.0 | pg_catalog | PL/pgSQL procedural language
timescaledb | 2.7.0 | public | Enables scalable inserts and complex queries for time-series data
(2 rows)
```
### Create a hypertable
Hypertables are the core of TimescaleDB. Hypertables enable TimescaleDB to work efficiently with time-series data. Because TimescaleDB is PostgreSQL, all the regular PostgreSQL tables, indexes, stored procedures and other objects can be created alongside your TimescaleDB hypertables. This makes creating and working with TimescaleDB tables similar to regular PostgreSQL.
#### Hypertables and chunks
Hypertables and chunks make storing and querying times-series data fast at petabyte scale.
TimescaleDB automatically partitions time-series data into chunks, or sub-tables, based on time and space. You can configure chunk size so that recent chunks fit in memory for faster queries.
A hypertable is an abstraction layer over chunks that hold the time-series data. Hypertables enable you to query and access data from all the chunks within the hypertable. You get all the benefits of automatic chunking for time-series data, alongside the simplicity of working with what looks like a standard, single PostgreSQL table.
Hypertables and chunks enable superior performance for shallow and wide queries, like those used in real-time monitoring. They are also good for deep and narrow queries, like those used in time-series analysis.
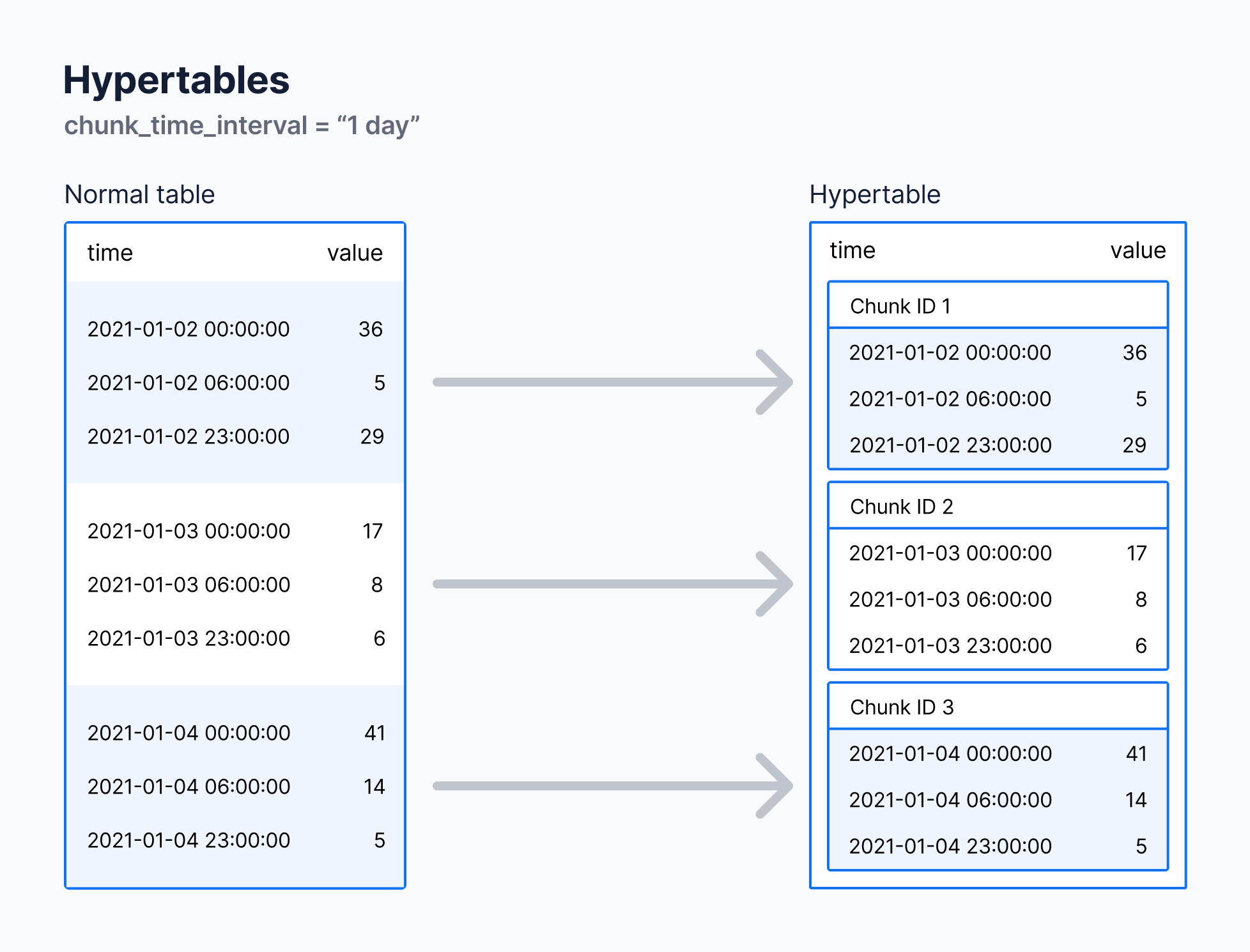
### Creating your first hypertable
1. Create a regular PostgreSQL table to store the real-time stock trade data using CREATE TABLE:
```
CREATE TABLE stocks_real_time (
time TIMESTAMPTZ NOT NULL,
symbol TEXT NOT NULL,
price DOUBLE PRECISION NULL,
day_volume INT NULL
);
```
2. Convert the regular table into a hypertable partitioned on the time column using the create_hypertable() function provided by TimescaleDB. You must provide the name of the table (stocks_real_time) and the column in that table that holds the timestamp data to use for partitioning (time):
```
SELECT create_hypertable('stocks_real_time','time');
```
3. Create an index to support efficient queries on the symbol and time columns:
```
CREATE INDEX ix_symbol_time ON stocks_real_time (symbol, time DESC);
```
### Compression
TimescaleDB includes native compression capabilities which enable you to analyze and query massive amounts of historical time-series data inside a database while also saving on storage costs. Additionally, all PostgreSQL data types can be used in compression.
Compressing time-series data in a hypertable is a two-step process. First, you need to enable compression on a hypertable by telling TimescaleDB how to compress and order the data as it is compressed. Once compression is enabled, the data can then be compressed in one of two ways:
* Using an automatic policy
* Manually compressing chunks
Enable TimescaleDB compression on the hypertable
To enable compression, you need to ALTER the stocks_real_time hypertable. There are three parameters you can specify when enabling compression:
* timescaledb.compress (required): enable TimescaleDB compression on the hypertable
* timescaledb.compress_orderby (optional): columns used to order compressed data
* timescaledb.compress_segmentby (optional): columns used to group compressed data
If you do not specify compress_orderby or compress_segmentby columns, the compressed data is automatically ordered by the hypertable time column.
#### Enabling compression on a hypertable
Use this SQL function to enable compression on the stocks_real_time hypertable:
```
ALTER TABLE stocks_real_time SET (
timescaledb.compress,
timescaledb.compress_orderby = 'time DESC',
timescaledb.compress_segmentby = 'symbol'
);
```
View and verify the compression settings for your hypertables by using the compression_settings informational view, which returns information about each compression option and its orderby and segmentby attributes:
```
SELECT * FROM timescaledb_information.compression_settings;
```
The results look like this:
```
hypertable_schema|hypertable_name |attname|segmentby_column_index|orderby_column_index|orderby_asc|orderby_nullsfirst|
-----------------+----------------+-------+----------------------+--------------------+-----------+------------------+
public |stocks_real_time|symbol | 1| | | |
public |stocks_real_time|time | | 1|false |true |
```
### Automatic compression
When you have enabled compression, you can schedule a policy to automatically compress data according to the settings you defined earlier.
For example, if you want to compress data on your hypertable that is older than two weeks, run this SQL:
```
SELECT add_compression_policy('stocks_real_time', INTERVAL '2 weeks');
```
Similar to the continuous aggregates policy and retention policies, when you run this SQL, all chunks that contain data that is at least two weeks old are compressed in stocks_real_time, and a recurring compression policy is created.
It is important that you don't try to compress all your data. Although you can insert new data into compressed chunks, compressed rows can't be updated or deleted. Therefore, it is best to only compress data after it has aged, once data is less likely to require updating.
Just like for automated policies for continuous aggregates, you can view information and statistics about your compression background job in these two information views:
Policy details:
```
SELECT * FROM timescaledb_information.jobs;
```
#### Manual compression
While it is usually best to use compression policies to compress data automatically, there might be situations where you need to manually compress chunks.
Use this query to manually compress chunks that consist of data older than 2 weeks. If you manually compress hypertable chunks, consider adding if_not_compressed=>true to the compress_chunk() function. Otherwise, TimescaleDB shows an error when it tries to compress a chunk that is already compressed:
```
SELECT compress_chunk(i, if_not_compressed=>true)
FROM show_chunks('stocks_real_time', older_than => INTERVAL ' 2 weeks') i;
```
#### Verify your compression
You can check the overall compression rate of your hypertables using this query to view the size of your compressed chunks before and after applying compression:
```
before compression | after compression
--------------------+-------------------
452 MB | 40 MB
(1 row)
```